Introduction to Artificial Neural Networks Part 2 - Learning
Welcome to part 2 of the introduction to my artificial neural networks series, if you haven’t yet read part 1 you should probably go back and read that first!Introduction
In part 1 we were introduced to what artificial neural networks are and we learnt the basics on how they can be used to solve problems. In this tutorial we will begin to find out how artificial neural networks can learn, why learning is so useful and what the different types of learning are. We will specifically be looking at training single-layer perceptrons with the perceptron learning rule.Before we begin, we should probably first define what we mean by the word learning in the context of this tutorial. It is still unclear whether machines will ever be able to learn in the sense that they will have some kind of metacognition about what they are learning like humans. However, they can learn how to perform tasks better with experience. So here, we define learning simply as being able to perform better at a given task, or a range of tasks with experience.
Learning in Artificial Neural Networks
One of the most impressive features of artificial neural networks is their ability to learn. You may recall from the previous tutorial that artificial neural networks are inspired by the biological nervous system, in particular, the human brain. One of the most interesting characteristics of the human brain is it’s ability to learn. We should note that our understanding of how exactly the brain does this is still very primitive, although we do still have a basic understanding of the process. It is believed that during the learning process the brain’s neural structure is altered, increasing or decreasing the strength of it’s synaptic connections depending on their activity. This is why more relevant information is easier to recall than information that hasn’t been recalled for a long time. More relevant information will have stronger synaptic connections and less relevant information will gradually have it’s synaptic connections weaken, making it harder to recall.Although simplified, artificial neural networks can model this learning process by adjusting the weighted connections found between neurons in the network. This effectively emulates the strengthening and weakening of the synaptic connections found in our brains. This strengthening and weakening of the connections is what enables the network to learn.
Learning algorithms are extremely useful when it comes to certain problems that either can’t be practically written by a programmer or can be done more efficiently by a learning algorithm. Facial recognition would be an example of a problem extremely hard for a human to accurately convert into code. A problem that could be solved better by a learning algorithm, would be a loan granting application which could use past loan data to classify future loan applications. Although a human could write rules to do this, a learning algorithm can better pick up on subtleties in the data which may be hard to code for.
Learning Types
There are many different algorithms that can be used when training artificial neural networks, each with their own separate advantages and disadvantages. The learning process within artificial neural networks is a result of altering the network's weights, with some kind of learning algorithm. The objective is to find a set of weight matrices which when applied to the network should - hopefully - map any input to a correct output. In this tutorial, the learning type we will be focusing on is supervised learning. But before we begin, lets take a quick look at the three major learning paradigms.- Supervised Learning
The learning algorithm would fall under this category if the desired output for the network is also provided with the input while training the network. By providing the neural network with both an input and output pair it is possible to calculate an error based on it’s target output and actual output. It can then use that error to make corrections to the network by updating it’s weights. - Unsupervised Learning
In this paradigm the neural network is only given a set of inputs and it’s the neural network’s responsibility to find some kind of pattern within the inputs provided without any external aid. This type of learning paradigm is often used in data mining and is also used by many recommendation algorithms due to their ability to predict a user's preferences based on the preferences of other similar users it has grouped together. - Reinforcement Learning
Reinforcement learning is similar to supervised learning in that some feedback is given, however instead of providing a target output a reward is given based on how well the system performed. The aim of reinforcement learning is to maximize the reward the system receives through trial-and-error. This paradigm relates strongly with how learning works in nature, for example an animal might remember the actions it’s previously taken which helped it to find food (the reward).
Implementing Supervised Learning
As mentioned earlier, supervised learning is a technique that uses a set of input-output pairs to train the network. The idea to provide the network with examples of inputs and outputs then to let it find a function that can correctly map the data we provided to a correct output. If the network has been trained with a good range of training data when the network has finished learning we should even be able to give it a new, unseen input and the network should be able to map it correctly to an output.There are many different supervised learning algorithms we could use but the most popular, and the one we will be looking at in more detail is backpropagation. Before we look at why backpropagation is needed to train multi-layered networks, let’s first look at how we can train single-layer networks, or as they’re otherwise known, perceptrons.
The Perceptron Learning rule
The perceptron learning ruleworks by finding out what went wrong in the network and making slight corrections to hopefully prevent the same errors happening again. Here’s how it works… First we take the network's actual output and compare it to the target output in our training set. If the network's actual output and target output don’t match we know something went wrong and we can update the weights based on the amount of error. Lets run through the algorithm step by step to understand how exactly it works.First, we need to calculate the perceptron’s output for each output node. As you should remember from the previous tutorial we can do this by:
output = f( input1 × weight1 + input2 × weight2 + … )
- or -
$o = f(\sum\limits_{i=1}^n x_iw_i)$Now we have the actual output we can compare it to the target output to find the error:
error = target output - output
- or -
E = t - oNow we want to use the perceptron’s error to adjust the weights.
weight change = learning rate × error × input
- or -
Δwi = r E xWe want to ensure only small changes are made to the weights on each iteration, so to do this we apply a small learning rate (r). If the learning rate is too high the perceptron can jump too far and miss the solution, if it’s too low, it can take an unreasonably long time to train.
This gives us a final weight update equation of:
weight change = learning rate × (target output - actual output) × input
- or -
Δwi = r ( t - o ) xiHere's an example of how this would work with the AND function...
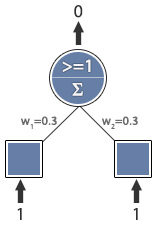
Learning rate = 0.1
Expected output = 1
Actual output = 0
Error = 1
Weight Update:
wi = r E x + wi
w1 = 0.1 x 1 x 1 + w1
w2 = 0.1 x 1 x 1 + w2
New Weights:
w1 = 0.4
w2 = 0.4
Expected output = 1
Actual output = 0
Error = 1
Weight Update:
wi = r E x + wi
w1 = 0.1 x 1 x 1 + w1
w2 = 0.1 x 1 x 1 + w2
New Weights:
w1 = 0.4
w2 = 0.4
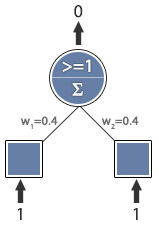
Learning rate = 0.1
Expected output = 1
Actual output = 0
Error = 1
Weight Update:
wi = r E x + wi
w1 = 0.1 x 1 x 1 + w1
w2 = 0.1 x 1 x 1 + w2
New Weights:
w1 = 0.5
w2 = 0.5
Expected output = 1
Actual output = 0
Error = 1
Weight Update:
wi = r E x + wi
w1 = 0.1 x 1 x 1 + w1
w2 = 0.1 x 1 x 1 + w2
New Weights:
w1 = 0.5
w2 = 0.5
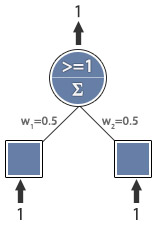
Learning rate = 0.1
Expected output = 1
Actual output = 1
Error = 0
No error,
training complete.
Expected output = 1
Actual output = 1
Error = 0
No error,
training complete.
Implementing The Perceptron Learning Rule
To help fully understand what’s happening let’s implement a basic example in Java.First, we initiate our network’s threshold, learning rate and weights. We could initiate the weights with a small random starting weight, however for simplicity here we'll just set them to 0.
double threshold = 1;
double learningRate = 0.1;
double[] weights = {0.0, 0.0};
double learningRate = 0.1;
double[] weights = {0.0, 0.0};
Next, we need to create our training data to train our perceptron. In this example our perceptron will be learning the AND function.
// AND function Training data
int[][][] trainingData = {
{{0, 0}, {0}},
{{0, 1}, {0}},
{{1, 0}, {0}},
{{1, 1}, {1}},
};
int[][][] trainingData = {
{{0, 0}, {0}},
{{0, 1}, {0}},
{{1, 0}, {0}},
{{1, 1}, {1}},
};
Now, we need to create a loop that we can break from later if our network completes a cycle of the training data without any errors. Then, we need a second loop that will iterate over each input in the training data.
// Start training loop
while(true){
int errorCount = 0;
// Loop over training data
for(int i=0; i < trainingData.length; i++){
System.out.println("Starting weights: " + Arrays.toString(weights));
}
while(true){
int errorCount = 0;
// Loop over training data
for(int i=0; i < trainingData.length; i++){
System.out.println("Starting weights: " + Arrays.toString(weights));
}
From here we can calculate the weighted sum of the inputs and get the output.
// Calculate weighted sum of inputs
double weightedSum = 0;
for(int ii=0; ii < trainingData[i][0].length; ii++) {
weightedSum += trainingData[i][0][ii] * weights[ii];
}
// Calculate output
int output = 0;
if(threshold <= weightedSum){
output = 1;
}
System.out.println("Target output: " + trainingData[i][1][0] + ", "
+ "Actual Output: " + output);
double weightedSum = 0;
for(int ii=0; ii < trainingData[i][0].length; ii++) {
weightedSum += trainingData[i][0][ii] * weights[ii];
}
// Calculate output
int output = 0;
if(threshold <= weightedSum){
output = 1;
}
System.out.println("Target output: " + trainingData[i][1][0] + ", "
+ "Actual Output: " + output);
The next step is to calculate the error and adjust the weights…
// Calculate error
int error = trainingData[i][1][0] - output;
// Increase error count for incorrect output
if(error != 0){
errorCount++;
}
// Update weights
for(int ii=0; ii < trainingData[i][0].length; ii++) {
weights[ii] += learningRate * error * trainingData[i][0][ii];
}
System.out.println("New weights: " + Arrays.toString(weights));
System.out.println();
int error = trainingData[i][1][0] - output;
// Increase error count for incorrect output
if(error != 0){
errorCount++;
}
// Update weights
for(int ii=0; ii < trainingData[i][0].length; ii++) {
weights[ii] += learningRate * error * trainingData[i][0][ii];
}
System.out.println("New weights: " + Arrays.toString(weights));
System.out.println();
Finally, break if a solution is found and close loop
// If there are no errors, stop
if(errorCount == 0){
System.out.println("Final weights: " + Arrays.toString(weights));
System.exit(0);
}
}
if(errorCount == 0){
System.out.println("Final weights: " + Arrays.toString(weights));
System.exit(0);
}
}
And if we put it all together…
package perceptron;
import java.util.Arrays;
public class PerceptronLearningRule {
public static void main(String args[]){
double threshold = 1;
double learningRate = 0.1;
// Init weights
double[] weights = {0.0, 0.0};
// AND function Training data
int[][][] trainingData = {
{{0, 0}, {0}},
{{0, 1}, {0}},
{{1, 0}, {0}},
{{1, 1}, {1}},
};
// Start training loop
while(true){
int errorCount = 0;
// Loop over training data
for(int i=0; i < trainingData.length; i++){
System.out.println("Starting weights: " + Arrays.toString(weights));
// Calculate weighted input
double weightedSum = 0;
for(int ii=0; ii < trainingData[i][0].length; ii++) {
weightedSum += trainingData[i][0][ii] * weights[ii];
}
// Calculate output
int output = 0;
if(threshold <= weightedSum){
output = 1;
}
System.out.println("Target output: " + trainingData[i][1][0] + ", "
+ "Actual Output: " + output);
// Calculate error
int error = trainingData[i][1][0] - output;
// Increase error count for incorrect output
if(error != 0){
errorCount++;
}
// Update weights
for(int ii=0; ii < trainingData[i][0].length; ii++) {
weights[ii] += learningRate * error * trainingData[i][0][ii];
}
System.out.println("New weights: " + Arrays.toString(weights));
System.out.println();
}
// If there are no errors, stop
if(errorCount == 0){
System.out.println("Final weights: " + Arrays.toString(weights));
System.exit(0);
}
}
}
}
Perceptron learning rule sourceimport java.util.Arrays;
public class PerceptronLearningRule {
public static void main(String args[]){
double threshold = 1;
double learningRate = 0.1;
// Init weights
double[] weights = {0.0, 0.0};
// AND function Training data
int[][][] trainingData = {
{{0, 0}, {0}},
{{0, 1}, {0}},
{{1, 0}, {0}},
{{1, 1}, {1}},
};
// Start training loop
while(true){
int errorCount = 0;
// Loop over training data
for(int i=0; i < trainingData.length; i++){
System.out.println("Starting weights: " + Arrays.toString(weights));
// Calculate weighted input
double weightedSum = 0;
for(int ii=0; ii < trainingData[i][0].length; ii++) {
weightedSum += trainingData[i][0][ii] * weights[ii];
}
// Calculate output
int output = 0;
if(threshold <= weightedSum){
output = 1;
}
System.out.println("Target output: " + trainingData[i][1][0] + ", "
+ "Actual Output: " + output);
// Calculate error
int error = trainingData[i][1][0] - output;
// Increase error count for incorrect output
if(error != 0){
errorCount++;
}
// Update weights
for(int ii=0; ii < trainingData[i][0].length; ii++) {
weights[ii] += learningRate * error * trainingData[i][0][ii];
}
System.out.println("New weights: " + Arrays.toString(weights));
System.out.println();
}
// If there are no errors, stop
if(errorCount == 0){
System.out.println("Final weights: " + Arrays.toString(weights));
System.exit(0);
}
}
}
}
Bias units
In our last example we set our threshold to 1, this means our weighted input needs to equal or exceed 1 to give us an output of 1. This is okay when learning the AND function because we know we only need an output when both inputs will be set, allowing (with the correct weights) for the threshold to be reached or exceeded. In the case of the NOR function however, the network should only output 1 if both inputs are off. This means if we have a threshold of 1 there isn’t a combination of weights that will ever make the following true,x1 = 0
x2 = 0
1 <= x1w1 + x2w2
x2 = 0
1 <= x1w1 + x2w2
There’s a simple fix to this though, a bias unit. A bias unit is simply a neuron with a constant output, typically of 1. Bias units are weighted just like other units in the network, the only difference is that they will always output 1 regardless of the input from the previous layer, this is where they get their name! So why are they important? Bias inputs effectively allow the neuron to learn a threshold value. Consider our previous equation, with a bias input (x0) added we can change it to,
x0 = 1
x1 = 0
x2 = 0
1 <= x0w0 + x1w1 + x2w2
x1 = 0
x2 = 0
1 <= x0w0 + x1w1 + x2w2
Now we can satisfy that equation. You can try this yourself by updating your perceptron training set to train for the NOR function. Just add a bias input to the training data and also an additional weight for the new bias input. Here is the updated code:
// Init weights
double[] weights = {0.0, 0.0, 0.0};
// NOR function training data
int[][][] trainingData = {
{{1, 0, 0}, {1}},
{{1, 0, 1}, {0}},
{{1, 1, 0}, {0}},
{{1, 1, 1}, {0}},
};
double[] weights = {0.0, 0.0, 0.0};
// NOR function training data
int[][][] trainingData = {
{{1, 0, 0}, {1}},
{{1, 0, 1}, {0}},
{{1, 1, 0}, {0}},
{{1, 1, 1}, {0}},
};
To be continued…
Hopefully you should now have a clearer understanding about the types of learning we can apply to neural networks and the process in which a simple, single layer perceptrons can be trained. In the next tutorial we will be learning how to implement the back propagation algorithm and why it's needed when working with multi-layer networks.Author

I'm a developer from the UK who writes about technology and startups. Here you'll find articles and tutorials about things that interest me. If you want to hire me or know more about me head over to my about me page